Success Assured®
This short 2-minute video is a quick overview on Multi-Dimensional Trade Studies:
(but please read the rest of this page for more details and some interesting side stories)
A Related Story...
A team engineering a consumer product needed to bring in some experts from another discipline to fill in a missing relationship in their trade-off model. The three experts "knew" the relation being asked for, but didn't have an equation for it or hard data.
Undeterred, the team did as they have been taught, and asked the experts what happened as the values went to zero and as the values got very large, giving them the general shape of the curve. Then they asked if the experts knew some specific values to help position that curve, which the experts did.
They then showed the experts the resulting multi-dimensional relation in a Trade-Off Chart to validate that it was consistent with their understanding. As they moved through that corner of the trade space, one of the experts said, "Wait, go back! Yeah, there, where the lines cross. John, look at that... that explains why we have to adjust our rules of thumb in some cases... the lines cross, meaning the direction of the normal effect actually reverses at that point." Much discussion ensued as they worked out how they could leverage this new understanding.
Note that this deeper understanding came from simply making visible that multi-dimensional trade space. We were just showing them the knowledge we had pulled from their own heads, but they had no way to "see" it.
And that was just from the single relation they were providing. The insight gained by the larger team from seeing the multi-dimensional trade space for the multiple relations covering multiple disciplines was far greater, as that allowed them to see knowledge that none of them previously had in their heads (though it was in their collective heads).
ABOUT THE SITUATION
The Many Dimensions of "Multi-Dimensional"
There is a little more to it than just handling multi-dimensional trades:
-
Multi-Relational – there are typically multiple independent relationships that drive the sensitivities and the upper and lower limits between the trade-offs
-
Multi-Discipline – there are typically several different disciplines (areas of expertise) that need to be included in the trade-off analyses
-
Multi-Fidelity – there are typically some things known fairly accurately while others are rough estimates, projections, or rules of thumb; or would take too long to compute at anything other than a lower fidelity
-
Multi-Study – there is often need to leverage past studies that may have had slightly different assumptions or otherwise need to be padded or adjusted for use
-
Multi-Tool – there are often specialty tools that need to be leveraged in the trade-off analyses due to the variety of disciplines, fidelities, etc.
-
Multi-Dimensional – and, of course, very rarely are the resulting trades just between two decisions; an elaborate decision space of 10 or more competing issues is more common
Worse, trade studies are often done as part of a learning & innovation process, meaning:
-
Multi-Timing – there may be a series of due dates for different decisions and different levels of convergence of those decisions
-
Multi-Perspective – different people from different disciplines may be leveraging the same trade study from different perspectives, wanting to see different things
-
Multi-Result – the same trade study may be leveraged to deliver different results to support those different due dates and different perspectives, not just one answer
-
Multi-Input/Assumption/Requirement – the team is learning and innovating and thus the inputs to the trade study may be changing, forcing the trade study to evolve with the rest of the project
The all-too-common response to all those "multi-" demands is to do isolated simpler studies and then to rely on human intuition to make the larger trade-offs. And although human intuition does amazingly well considering the complexity, missed issues and mis-estimated interactions is the norm, not the exception. 65-75% of our engineering capacity is consumed by rework from the bad decisions based on trade-off analyses that rely too heavily on human intuition and not heavily enough on making the trades and interactions clearly visible to those decision makers.

Another Related Story...
A team had spent the prior three weeks trying to find a solution in a new part of the design space; they weren't really certain that it was possible. So, as they completed their new trade space model in our training workshop, they were anxious to pull up a Chart and see what was possible in that space.
"Hmmmf, as I feared, there's nothing there."
But it was a high-dimensional design space and we pointed out that their 3D chart is just slices through that multi-dimensional space. If the solution is thin, it could lie between the slices. The Chart is the right tool for seeing sensitivities and limits of a design space, but not for determining all that's possible in a multi-dimensional space. The right tool for that is the Trade-Off Solver.
Fortunately, there's a button in every Trade-Off Chart that will generate the equivalent Trade-Off Solver; and clicking it revealed there was indeed a solution where they wanted. We then used the Solver to narrow to that part of the design space and from that Solver generate a Trade-Off Chart showing 3D slices through that solution space.
It was then clear why their many guessed solutions the past 3 weeks had missed the solution space: it was a very thin sliver through the vast multi-dimensional design space.
ABOUT THE HUMAN LIMITATIONS
We're very good at Three
-
The 3D visual processing in our visual cortex consumes a significant portion of our brains.
-
Our ability to process and deeply understand 3D visual data is remarkable.
-
Similarly, our ability to project 1D or 2D visuals to/from 3D space is outstanding.
-
But ask us to visualize a 4D space and... well... we got nuthin', so to speak.
-
Ask us to project a 4D space into 3D so that we can see it (like we do with 3D space into 2D so that we can put it on paper), and again we struggle.
-
And yet, most every complex problem our teams are working on have far more than 3 dimensions.
So, a key challenge is how to get the results of a 10+ dimensional trade study into our brains so that we can gain the insight we need to make the right decisions. And closely related, how to enable a team of people with different expertise to collaborate in that decision-making regarding that 10+ dimensional trade space.
There are other things we humans tend to be weak at which impact effective creation and use of multi-dimensional trade studies:
-
Uncertainty. We tend to want to insert a single value best guess. What we should be doing is looking at the full set of possibilities and make sure we're okay with the worst-case.
-
Statistics. We are notoriously bad at properly interpreting probabilities and statistics.
-
Aggregation. It is well-known, widely-observed that if we are estimating times for doing tasks that are a few hours or a few days, then we tend to be fairly accurate; but if estimating tasks that take a few weeks or months, then we tend to be quite inaccurate as we lose sight of the details and the interactions. A similar pattern happens when estimating the totals for systems of subsystems of components, or any other aggregation.
-
Combinatorics. We deal well with linear relationships; not so well with exponential ones. For example, if offered $10,000 per day for a month, or a penny the first day, and then double that the next day, and so on for that same month, which would you choose? Most human intuition would take the $10,000 per day and net $310,000. Those who have worked through the math would choose the doubling penny, and net $21,474,836.47.
Human intuition and innovation is incredibly powerful and should be leveraged in all our analysis and decision-making. But it also has its weaknesses that we should be well-aware of and use our visualization and analysis tools to back-fill, adjust, and auto-correct.
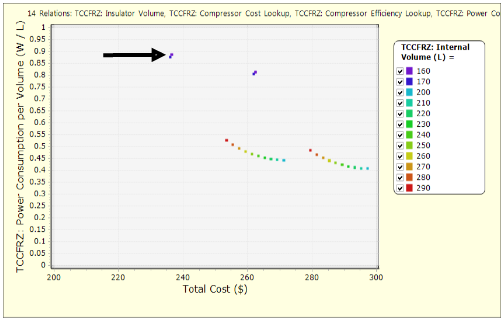
ABOUT OUR SOLUTION
Successfully Navigating Multi-Dimensional Trade Spaces
The key challenge is not simply modeling and computing the trade study; the key challenge is effectively communicating the multi-dimensional trade space to the team of people with different areas of expertise such that they can effectively collaborate, identify flaws, innovate, and converge efficiently to consensus decisions based on concrete knowledge.
Our solution starts with a Causal Map tool for capturing the structure of the multi-dimensional trade space in the form of the Decisions that need to be made connected by Relations that capture the knowledge of how those Decisions impact each other (how they trade-off) at whatever level and fidelity is easiest for the experts in that particular area to provide. That Causal Map is initially a map of what is known and not known, such that it can drive the learning effort in the most efficient way.
Once the knowledge gaps have been closed (the Relations have been filled in), then the Causal Map has been transformed into a computable Decision Map from which our software can generate a variety of Trade-Off Charts and Solvers, which together give visibility to the limits and sensitivities of the multi-dimensional trade space.
By allowing the team to easily move between those three different visual models of the trade space, making adjustments in each to what 2D or 3D slices through that multi-D space they want to see, they are able to gain a much deeper understanding. To help communicate the multi-dimensional data into our 3D brains, our software leverages the next strongest human sense after the visual cortex: our hand-eye coordination. By manipulating the Chart's animators to show their eyes different slices through the space, we are able to generate an intuitive feel for those multi-dimensional interactions.
The visual modeling tools support in-depth reviews and audits by people from different disciplines with different expertise, resulting in fewer errors, better continuous improvement, and more innovation:
-
When presented a Chart or Solver, if the reviewer wants to understand what those computations are based on, they are always just one button away from a visual Trade-Off Map showing them the underlying Decision Map and how that multi-dimensional trade space is being mapped into the Chart. That makes challenging and auditing the logic of your decision-making quick and easy, and continuous improvement efficient.
-
Further, the reviewers can use that same Trade-Off Map to quickly reconfigure the Charts to show them the limits and sensitivities the way they want to see those. The right 3D slices through the multi-dimensional space may be different for those with different expertise or different focus.
-
If certain Relations are of concern, it is easy to substitute in an alternative and see the impact. If the impact is small, then the team may gain comfort their decisions are resilient to that uncertainty; if the impact is large, then the team may have the justification they need to close that knowledge gap.
-
That prior capability is also used to deal with multi-fidelity situations; you can potentially have substitute Relations with different levels of fidelity as appropriate for the situation (e.g., how early in the decision process; availability of knowledge; time constraints for the computations)
-
leverage strong typing, units of measure, and dimensional analysis to automate error-checking of the end-user formulations, further reducing mistakes, and simplifying “end user development” by automating some of the most error-prone work (like converting between different units of measure)
-
leverage the natural 2D mathematical notation for equations, along with use of visual cues to aid in human reading and auditing of those equations to further reduce errors and improve collaboration and analysis
Our software's Set-Based computational engine enables superior optimization within the entire trade space (all infinite points):
-
Enables human-in-the-loop optimization that corresponds better to how people need to make decisions, particularly regarding how customers will react
-
Exposes worst-case limits and safe vs. unsafe regions for making wise decisions, even in the face of uncertainty
-
Avoids models that miss key anomalies or catastrophic failure points
-
Enables "eliminate the weak" optimization, which in turn enables efficient convergent decision-making
Yet Another Related Story...
The Army (ARDEC) was designing an appropriate unmanned air vehicle (UAV) for a variety of missions that need the UAV to as quickly as possible travel to a target area (that might be 10km away), and then scan that area (that might be 5 km²) to detect either human or vehicular activity (with 80-99% probability of detection). And they might need that during either day or night. At the same time, they want to minimize the probability of their UAV being spotted, and if spotted minimize the likelihood of being shot down. And of course, they want to minimize weight (since they have to lug these around) and cost. That is a fairly typical multi-dimensional trade-off.
To simplify that, they developed a value model that allowed them to reduce it to a 2-dimensional trade-off between cost and the weighted sum of the value numbers assigned to each of the dimensions above. We captured that model into Success Assured® and computed the full trade space (all infinite points) as shown below:
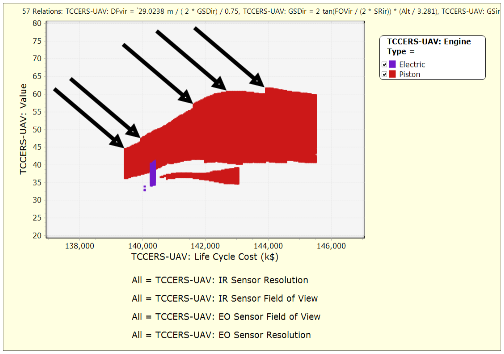
Or the same trade space can be colored by Altitude instead of Engine Type:

And to the extent the stakeholders are fully satisfied with the value model accurately reflecting their preferences, they can simply choose which of those points in that design space is most desirable and then work backward to the corresponding design decisions.
Or they can peel off the value model and look at the multi-dimensional trade space underneath. For example, consider the customer interest Probability of Detecting a Human. In this Chart you can see the sensitivity to the Ground Sample Distance (which makes sense that would need to be at the granularity of human dimensions):

Of course, you can't directly control Ground Sample Distance, so better may be to instead Chart versus the related Sensor Decisions that you can control, yielding this Chart. It shows that as you increase Sensor Resolution, it allows you to operate at a higher Altitude. Changing Sensor Field of View using the Animator moves these curves, but will have its own trades...

To see the trades with the Sensor Field of View, you can pull in additional Relations that connect it, the Altitude, and the Ground Swath through Ground Coverage Rate to the customer interest Time to Cover 5km², as shown in this Chart. As you Animate the EO Sensor's Resolution and Field of View decisions, you can see how it impacts how much Time to Cover and the Probability of Detection as you vary Cruise Velocity.
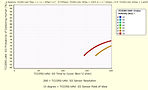
Three Layers of Reusable Trade Study Knowledge
A tremendous amount of valuable knowledge is generated in most trade study efforts. And given the significant effort involved, it is a waste if that knowledge is not easily reused. The key to reuse is that the generic reusable knowledge needs to be separate from the highly situation-specific knowledge. And the mechanisms need to do that automatically – it cannot be an extra effort to make the knowledge reusable. Rather, the natural flow of building the knowledge to get your work done, should capture it in a resuable format and organization. If making it reusable requires extra work, it will rarely be done.

Decision Maps
A Decision Map is a collection of related Decisions and Relations that together connect the decisions you can directly control to the customer interests you want to satisfy. The Relations capture the critical knowledge from the different disciplines.

Charts & Solvers
The Charts and Solvers capture how best to see the sensitivities and limits of the trade space and how best to optimize your competing decisions within that trade space to best satisfy your customers (all the stakeholders). From any Chart or Solver, you're always just one click away from the underlying Decision Map that it was computed from.
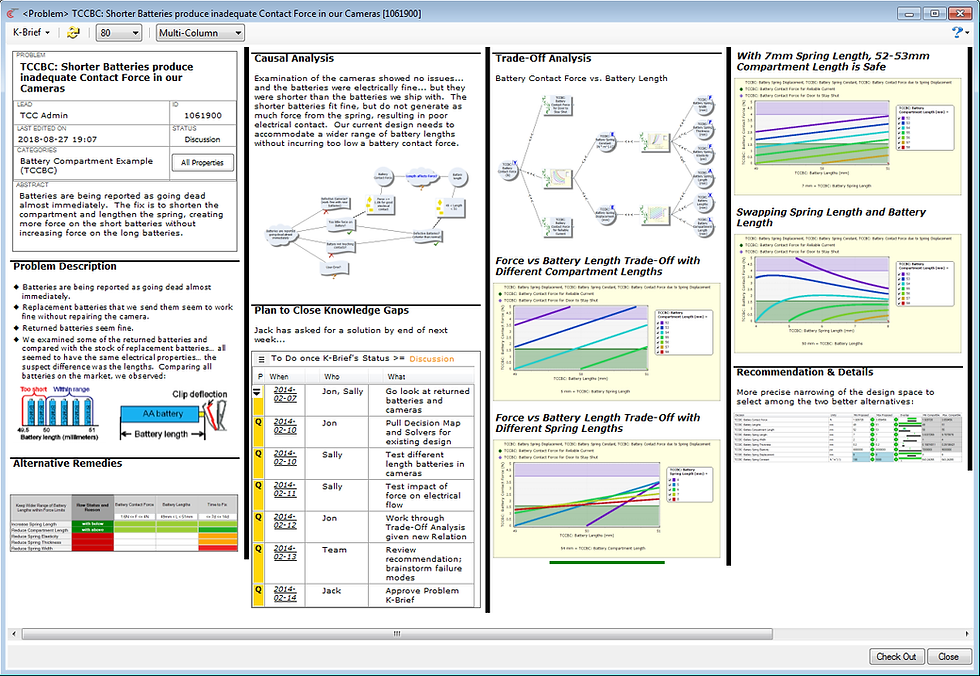
K-Briefs
The Project, Problem, and similar K-Briefs tell the story of how the Charts and Solvers are being used to make the required decisions, based on the underlying knowledge. That may include identification of gaps in that knowledge that should be closed prior to further converging those decisions.

Contact Us
If you have questions, would like a demo, or would like to schedule us to visit you, please email us at Answers@TargetedConvergence.Com. Alternatively, you may call us at 1-888-LRN-FRST (1-888-576-3778). Either way, we'll route you to the right person.
Or if you prefer, you can send email direct to:
-
Our Sales Team at Sales@TargetedConvergence.Com.
-
Our On-the-Job Coaching Team at Mentors@TargetedConvergence.Com.
-
Our Software Support Team at Support@TargetedConvergence.Com.
-
Our Website Team at Webmaster@TargetedConvergence.Com.
-
Our Accounting Team at Accounting@TargetedConvergence.Com.
-
Our Human Resources Team at HR@TargetedConvergence.Com.
After 10 years in Carrollton, we have moved a few miles south to the prestigious Las Colinas region of Irving, TX:
Targeted Convergence Corporation
320 Decker Dr #254-03
Irving, TX 75062-3999
You can visit (and Like! or Follow) us at our official pages on LinkedIn and Google Maps.
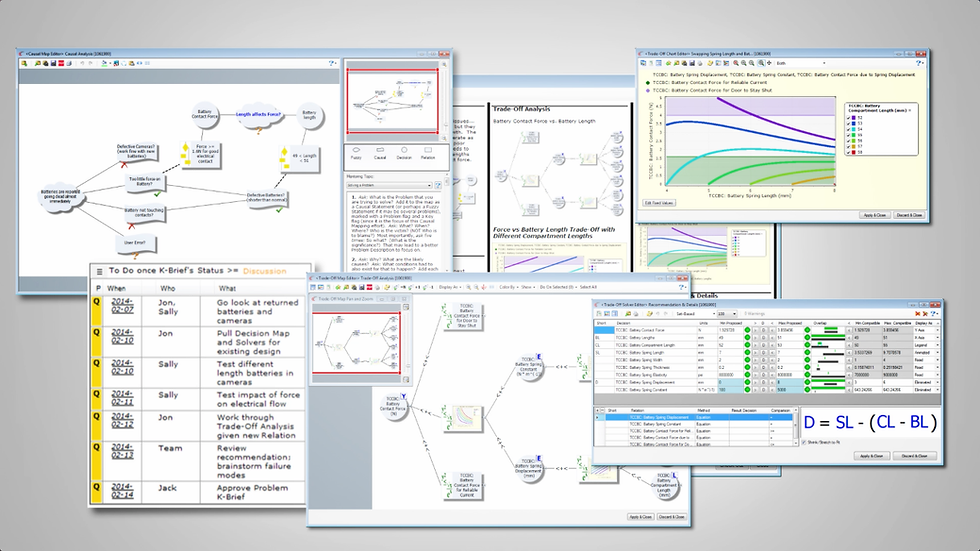